Imagine an app that feels like it thinks along with you, rather than for you. Instead of simple automation, it selectively offloads tasks requiring significant mental effort—the ones that slow you down. That’s a well-known concept in UX called “cognitive offloading,” usually referring to intuitive design of the type that Steve Krug wrote about in Don’t Make Me Think. But a few days ago, product designer Tetiana Sydorenko published an interesting article titled AI and cognitive offloading: sharing the thinking process with machines, in which she describes a model of thinking about the process of cognition itself and how it applies to UX design.
Thinking outside the brain’s boundaries
More specifically, she’s talking about where cognition is located. External mind theories hypothesize that cognition isn’t confined to an individual mind, but rather is more like an emergent property arising from a network of humans, the tools they use, and the artifacts they keep, passing down knowledge from prior generations. In this case the externalized mind takes the form of distributed cognition, as anthropologist Edwin Hutchins described it in his 1996 book Cognition in the Wild.
Hutchins observed that crew members of a navy ship navigated their way across the ocean as a team so integrated with each other that no individual crew member knew, or in fact needed to know, the entire route themselves. He pointed to each crew member functioning as a cognitive resource for each other, as well as the tools that they relied upon constantly such as a slide rule.
She frames Hutchins’s thesis in this way:
Hutchins proposed that to truly understand thinking, we must shift our focus. Rather than isolating the mind as the primary unit of analysis, we should examine the larger sociotechnical systems in which cognition occurs. These hybrid systems blend people, tools, and cultural artifacts, creating a web of interactions that extend far beyond any single individual.
Though Hutchins published his book in the mid-90’s, Sydorenko points out that the advancement of AI is ” revolutionizing the way we think and work by transforming how cognitive processes are distributed. Computers and AI systems take on repetitive and tedious tasks — like calculations, data storage, and searching — freeing humans to focus on creativity and problem-solving. But they don’t just lighten our mental load; they expand and enhance our cognitive abilities, enabling us to tackle problems and ideas in entirely new ways. Humans and computers together form powerful hybrid teams.”
Distributed Cognition in the Wild
Sydorenko then walks readers through a few examples of apps that she finds to particularly excel at using AI (and the AI we’re talking about is machine learning, more specifically generative AI, throughout) to manage cognitive offloading.
Probably the best example of distributed cognition in the list for me is Braintrust, an app designed for professional networking that uses AI to help employers create job listings.
Braintrust exemplifies how machine learning can act as a cognitive partner within a distributed system. By automating the creation of job descriptions, the platform reduces cognitive load while enabling collaboration between users and AI. This approach allows employers to focus on tailoring job roles while the AI handles the repetitive groundwork It also highlights the power of distributed cognition: tasks are shared across humans, machines, and tools to produce results more efficiently and effectively than any one component could achieve alone.
The common theme throughout the apps that Sydorenko visits on her tour is cognitive offloading, of course, and apart from Braintrust (professional networking) she walks us through the Craft app (note-taking and idea-generating), Red Sift Radar, (internet security) Relume (sitemap and wireframe generation), Airtable (business apps), Jasper (marketing), and June (product analysis).
These tools exemplify “cognitive augmentation,” a term that captures their ability to enhance and extend human thought processes, which I think is more accurate than “AI-assisted productivity” or something along those lines, because it gets at cognitive distribution while also suggesting a more dynamic interaction between human cognition and AI, now and in the future.
Backtracking a bit
But I want to step back and take a look at a couple of journal articles Sydorenko links in her piece, where she says “That was the ’90s. Today, modern information technologies, especially AI, are revolutionizing the way we think and work by transforming how cognitive processes are distributed.”
The first article, AI-teaming: Redefining collaboration in the digital era, examines how AI technologies influence human decision-making and perception. Interestingly, it notes some ambivalence about AI’s role, suggesting that the integration of AI in decision-making is not universally received as a positive development. This nuance complicates the narrative of seamless cognitive offloading that Sydorenko presents.
The second article is Supporting Cognition with Modern Technology: Distributed Cognition Today and in an AI-Enhanced Future, and sticking with the “in the wild” metaphor (Hutchins, after all, spent a lot of time in Papua New Guinea in his early career), it’s a lot of backpack to unpack.
It reframes distributed cognition in a modern AI context, focusing heavily on cognitive offloading while largely omitting its anthropological origins (no mention of Hutchins), but it nevertheless offers a robust framework for understanding cognitive offload that deserves to be unpacked.
It also mentions some risks accompanying cognitive offloading that are relevant to this discussion:
In three experiments, Grinschgl et al. (2021b) observed a trade-off for cognitive offloading: while the offloading of working memory processes increased immediate task performance, it also decreased subsequent memory performance for the offloaded information. Similarly, the offloading of spatial processes by using a navigation device impairs spatial memory (i.e., route learning and subsequent scene recognition; Fenech et al., 2010). Thus, information stored in a technical device might be quickly forgotten (for an intentional/directed forgetting account see Sparrow et al., 2011; Eskritt and Ma, 2014) or might not be processed deeply enough so that no long-term memory representations are formed (cf. depth of processing theories; Craik and Lockhart, 1972; Craik, 2002). In addition to detrimental effects of offloading on (long-term) memory, offloading hinders skill acquisition (Van Nimwegen and Van Oostendorp, 2009; Moritz et al., 2020) and harms metacognition (e.g., Fisher et al., 2015, 2021; Dunn et al., 2021); e.g. the use of technical aids can inflate one’s knowledge. In Dunn et al. (2021), the participants had to answer general knowledge questions by either relying on their internal resources or additionally using the internet. Metacognitive judgments showed that participants were overconfident when they are allowed to use the internet. Similarly, Fisher et al. (2021) concluded that searching for information online leads to a misattribution of external information to internal memory.
To summarize: cognitive offloading carries the risk of forgetting how to navigate without Google maps, lose documents on Google drive that you were going to use for a blog post, being lazy about learning new skills because AI can do it for you, and make you cockier about your own abilities because you forgot how much AI assistance has helped you out.
But none of those risks have anyone reading this post, or indeed its author, right? Of course not,
I’m reminded of the lawyers who were sanctioned last year for using ChatGPT to write a legal brief for them, unaware that it cited case law for cases that don’t exist.
But on the other hand, Eleventh Circuit Judge Kevin Newsom wrote a piece last May about how generative AI can assist judges in finding insights on the “ordinary meanings” of words. That may sound bizarre, until you recall that the term “legalese” exists for a reason: legal practitioners can become so entrenched in writing and speaking about the law that they fail to notice how disconnected their language is from a normal conversation between human beings.
Large Language Models, of course, can help you to write in many voices and levels of jargon, making them incredibly useful for the kinds of apps Sydorenko describes. But does that mean distributed cognition provides the best design principles for apps of all kinds, at least when it comes to generative AI?
A Trusted Tour Guide
The risks associated with cognitive offloading may contribute, at least in part, to the falling public trust in AI. According to a 2023 global study, 61% of us are wary of trusting AI systems.
An article in Forbes citing that global study noted:
it isn’t just about trusting AI to give us the right answers. It’s about the broader trust that society puts in AI. This means it also encompasses questions of whether or not we trust those who create and use AI systems to do it in an ethical way, with our best interests at heart.
Those of us who use AI to create content need to remember that we’re both expecting trust and being expected to be trustworthy– while most of us don’t design AI directly, we’re nevertheless responsible for what we make with it.
In that sense, we’re both consumers and creators of the famous “black box” that AI, particularly generative AI, represents. I can’t see whether you write something with AI, so not only don’t I know if you can write, but I don’t know whether you fact-checked the content you’re sharing. In the other direction, if I’m writing with AI assistance, I don’t know why the AI is making the suggestions and changes that it does.
But my aim here is not to get bogged down in questions about trust– rather, it’s to suggest an alternative external cognition model for UX design that incorporates AI, and that’s what philosophers Andy Clark and David Chalmers called, in their famous 1998 paper, the Extended Mind.
Clark and Chalmers envisioned the extended mind as a personal notebook, a helpful assistant, a brainstorming partner. The extended mind, to them, is active, integrated, and indispensable.
“Now consider Otto,” they begin in a now-famous thought experiment:
Otto carries a notebook around with him every- in the environment where he goes. When he learns new information, he writes it down. When he needs some old information, he looks it up. For Otto, his notebook plays the role usually played by a biological memory. Today, Otto hears about the exhibition at the Museum of Modern Art, and decides to go see it. He consults the notebook, which says that the museum is on 53rd Street, so he walks to 53rd Street and goes into the museum. Clearly, Otto walked to 53rd Street because he wanted to go to the museum and he believed the museum was on 53rd Street. . . it seems reasonable to say that Otto believed the museum was on 53rd Street even before consulting his notebook. For in relevant respects the cases are entirely analogous: the notebook plays for Otto the same role that memory plays for [someone else]. The information in the notebook functions just like the information constituting an ordinary non-occurrent belief; it just happens that this information lies beyond the skin.
If Otto were a computer, we’d call the notebook his “external hard drive.” In such a case, there wouldn’t be much contention if you called the HDD part of the computer, especially if the two remained connected and the computer regularly “consults” the HDD for information.
Because it’s all just data, right? Well, no. In the extended mind, there is what Clark and Chalmers call “active coupling.” The relationship between the mind and external tools must be causal, active, and continuous. It needs to reliably augment mental functioning.
The difference between distributed cognition and extended mind could be summed up, at a very high level, in this way:
Feature | Extended Mind | Distributed Cognition |
Focus | Individual cognition + tools | Group cognition + social systems |
Cognitive unit | Individual + artifact | Network of agents, tools, and systems |
AI relationship | AI as a personal cognitive extension | AI as part of a socio-technical system |
Level of analysis | Personal (one user + one tool) | Systems-level (multi-agent, cultural) |
Emphasis | Integration of tools into self | Collaboration, social interaction |
But what does an app that uses extended mind as a basis for design, rather than distributed cognition, actually look like? Here’s an example:
Ada is an AI-powered health companion that assists users in assessing symptoms and managing health concerns. Its design reflects extended mind principles through:
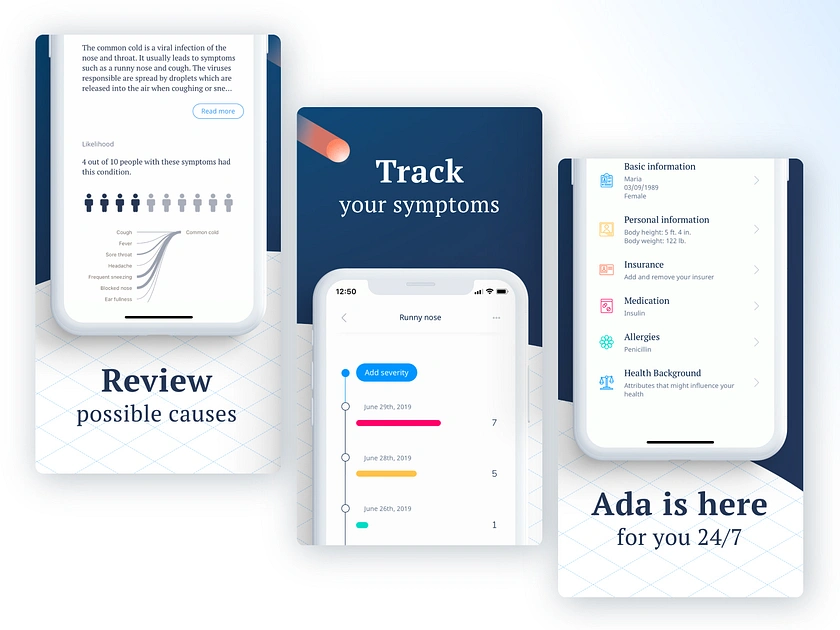
- User-Centric Interface: The app’s intuitive design ensures accessibility and ease of use, facilitating seamless integration into users’ health management routines.
- Interactive Symptom Assessment. Ada engages users in a conversational interface, asking personalized questions to evaluate symptoms, thereby acting as an extension of the user’s diagnostic reasoning.
- Personalized Health Insights. Based on user input, Ada provides tailored health information and guidance, supporting informed decision-making.
Now for some caveats: Ada isn’t an all-encompassing personal health app. It’s primarily designed to be a symptoms-checker. But by partnering with healthcare providers, it can be integrated into their systems as their “digital front door to services,” as the Ada site puts it, providing a first point of contact for questions about symptoms, available 24/7 (in fact, they say that 52% assessments were outside clinic opening hours). And this may not sound like a direct service to patients, but the site also proclaims that it “helped to redirect over 40% of users to less urgent care.” That’s compared to heading straight to the emergency room– it’s also available 24/7, but most people don’t want to go there if they have a viable alternative.
One more quote from the site:
A reputation built on trust
Ada’s fully explainable AI is designed to mimic a doctor’s reasoning. Our approach to safety, efficacy, and scientific scrutiny earns us the trust of clinicians and patients.
93% of patients said Ada asked questions that their doctor wouldn’t have considered
A person’s goal for using the Ada app isn’t cognitive augmentation– they don’t want to be doctors. They want to have access to information and recommendations that doctors can provide (but apparently, in some cases, don’t).
There are other differences between an app that evaluates your help systems and one that helps you write marketing copy, of course, and the most striking one is the stakes involved. Not to say that marketing copy isn’t important, but it’s important in the same way as an Uber is, relative to am ambulance– low risk vs. high risk.
Another difference is that because an app like Ada is healthcare-oriented and high risk, it carries a higher level of trust– which it has to earn. Healthcare apps may be more trustworthy in the eyes of the public than HR apps, but that will only sustain as long as the trust patients place in apps like Ada is justifiable. The context that makes Ada’s design work dramatically better with extended mind principles is the context that requires it to exist to begin with.
Two (complementary) paths forward
Hopefully by this point it will be clear that my intent is not to argue with Sydorenko’s post on distributed cognition for cognitive offload, but to “Yes, and” it. I think that both distributed cognition and extended mind hold great promise for the design of apps using AI, but which appropriate is more appropriate in a specific instance can depend on factors like “systemic vs. duality,” “collaboration vs. consultation,” and as basic as it sounds, “group vs. individual.”
But it’s also not as easy as “high stakes vs. everything else.” To illustrate that idea, I’ll toss out an example of an app that’s high stakes, healthcare-related, and best served by the distributed cognition model: PulsePoint.
PulsePoint is an app that tracks cardiac emergencies, such as heart attacks, and notifies nearby users who are trained in CPR to provide assistance. It exemplifies distributed cognition in the follow ways:
- It relies on a network of participants: 911 dispatchers, PulsePoint users, and emergency responders. Each plays a specific role in responding to cardiac emergencies. This shared cognitive load mirrors the distributed cognition model, where tasks are spread across multiple agents to achieve a collective goal.
- The app uses external tools (e.g., smartphones) to provide real-time alerts and locate Automated External Defibrillators (AEDs). These tools, combined with the users’ actions, form a socio-technical system.
- The system builds on past actions (e.g., training users in CPR, mapping AED locations) to support real-time decision-making during emergencies.
I’m planning to write more on the design needs of healthcare-related apps in the future, but for now, I hope Pulsepoint serves an example of how members of a collaborative system can work together to achieve amazing results via distributed cognition that otherwise would not have been possible.
That’s what “emergent property” means: combining to make something bigger than we could have achieved alone.
Both distributed cognition and the extended mind remind us that no matter the approach, our tools and networks amplify what we can achieve. Sometimes it takes a village, and sometimes it takes a friend. I know how corny that sounds, but sometimes it’s true.