My review of the mobile app, CBP One™: The Border in Your Pocket, considered factors in the development of CBP One’s facial recognition engine, the Traveler Verification Service (TVS), that render it unsuitable for CBP One’s current usage in collecting information from migrants at the border.
This post takes a closer look at how CBP represents its usage of AI Facial Recognition Technology (FRT), and why that representation almost never seems to acknowledge CBP One.
The problem with trying to nail down issues with CBP One’s use of FRT is the extent to which TVS’s use in CBP One is simply left out of the discussion. Here’s an example:
The Statement for the Record on Assessing CBP’s Use of Facial Recognition Technology, delivered on July 27, 2022, described the impressive benefits of the Traveler Verification Service, or TVS. TVS is the facial recognition engine used by the CBP One mobile application, along with many other applications. In fact, the statement said, “CBP developed TVS to be scalable and seamlessly applicable to all modes of transport throughout the travel continuum,” including the Global Entry Trusted Traveler Program:
CBP’s biometric facial comparison technology is integrated in all CBP’s legacy Global Entry kiosks, reducing kiosk processing time by 85 percent and CBP plans to deploy new Global Entry Touchless Portals at other locations around the country. These new Portals also utilize secure biometric facial comparison technology, are completely touch-free, and decrease processing time required by the biometric facial comparison technology by 94 percent to approximately 3.5 seconds per traveler.
Statement for the Record on Assessing CBP’s Use of Facial Recognition Technology
Given that most of the complaints about the app’s functionality have concerned its use of FRT, specifically demographic bias they attribute to it, you’d think it would be a simple task to learn what technology the app uses, then look up evaluations of that technology as it used in CBP One, as well as “all modes of transport throughout the transport continuum.” But it’s not that simple– and TVS is anything but “scalable and seamlessly applicable” to the “mode of transport” involving undocumented migrants attempting to use a mobile app to enter the country.
When reading a description of CBP’s AI facial recognition technology that fails to mention CBP One, you can only note its conspicuous absence. However, the stories told by CBP about its use of FRT say a lot about CBP One, even while not saying anything about it. CBP One is sort of the illegitimate child of the Department of Homeland Security.
In the original CBP One post I walked through the mobile app’s development and how the CBP One app itself, as well as its documentation, describe an app that CBP One was envisioned to become rather than the one it did.
But while time stopped within the app, it has continued to move forward in the rest of the world, including in the DHS’s use of AI FRT. Here are some specific clues of how CBP One has been left in the digital dust by its developers:
- The degree to which it goes unacknowledged that CBP One uses the same facial recognition engine as the other “modes of transport.”
- The lack of prior quality assessment performed on TVS as it is used in CBP One. As Lizzie O’Leary, host of Slate’s TBD podcast, said on the “Seeking Asylum Via App” episode, “migrants using CBP One are, in effect, beta testers.”
- Evaluations by NIST of facial recognition algorithms, including the NEC-2 and NEC-3 algorithms used by CBP, that speak to a number of factors negatively affecting the algorithm’s chances of avoiding false negatives in a 1-1 comparison for purposes of verification– but are apparently going ignored in favor of the algorithm’s performance on detecting false positives (aka, imposters).
- The implication, conspicuous by its omission, that CBP One is a use case demonstrating artificial intelligence functioning in a way we fear the most– making life-changing decisions without the requirement of direct human involvement, at any point in the process.
AI inventoried
According to the DHS AI Use Case Inventory, CBP uses AI Facial Comparison technology in the form of the Traveler Verification Service, or TVS. Its State of System Development Life Cycle is listed as “Operation and Maintenance,” and that it functions by creating image galleries “from images captured during previous entry inspections, photographs from U.S. passports and U.S. visas, and photographs from other DHS encounters.”
Interestingly, this description doesn’t apply to CBP One, which uses photos taken by immigrants’ cell phones. For that matter, it doesn’t mention CBP One at all. The inventory item for TVS describes a traveler “encountering a camera connected to TVS,” and migrants don’t typically “encounter” their phone cameras, nor are those cameras exactly “connected to TVS.”
CBP One is mentioned in the entry on “Use of Technology to Identify Proof of Life,” aka “Liveness Detection,” described as utilizing Machine Vision as its AI technique. Its State of System Development Cycle is listed as “Development and Acquisition,” which is interesting given that liveness detection has been in use in the CBP One app for at least two years now. Liveness detection AI is used in CBP One to “reduce fraudulent activity, primarily for use within the CBP One app.”
The description of Liveness Detection doesn’t mention TVS, though it does contain the boilerplate language about how CBP One “is designed to provide the public with a single portal to a variety of CBP services. It includes different functionality for travelers, importers, brokers, carriers, International Organizations, and other entities under a single consolidated log-in, and uses guided questions to help users determine the correct services, forms, or applications needed”– none of which is relevant to liveness detection in particular.
Your average American citizen could read this list and easily conclude that TVS is something that makes international travel easier and more expedient, while Liveness Detection is something in an app for immigrants to tell if they’re fraudulently trying to enter the country. But in fact, TVS is used by DHS for facial recognition/comparisons broadly (including Global Entry as mentioned above, TSA PreCheck, and commercial airline apps developed by Aiside), and both TVS and Liveness Detection are used in CBP One.
This matters because members of Congress are not especially different from your average American citizen. Several of them, including my state senator Roger Marshall, have (rightly, IMO) expressed grave concerns regarding the TSA’s use of FRT. Their May 2 letter to Chuck Schumer and Mitch McConnell read, in part,
Once Americans become accustomed to government facial recognition scans, it will be that much easier for the government to scan citizens’ faces everywhere, from entry into government buildings, to passive surveillance on public property like parks, schools, and sidewalks.1
Here’s the letter from 14 senators slamming TSA facial recognition in airports
And yet Marshall, at least, has been on a campaign to stop “unvetted” migrants from boarding domestic flights if their identities have been verified using CBP One.
So on the one hand he’s concerned about facial recognition working too well (else why would the government employ it everywhere, and why would it be so damaging if it did?), and on the other hand, he’s attempting to legislate airlines out of transporting migrants because the Traveler Verification Service (TVS) used in both scenarios doesn’t work not well enough. Else why complain that migrants are allowed “to enter our country and then board airlines free of charge without proper I.D. or vetting”? By failing to recognize that TVS is used in both CBP One and TSA facial recognition, he’s effectively trying to legislate for– and against– the same thing.
Facial Liveness might not be used on American citizens by the government, but iProov’s Flashmark technology is used for “Liveness Detection” in many applications, with use cases including onboarding, identity recovery, and multi-factor authentication. Its page on digital identity boasts:
According to the World Bank, countries extending full digital identity coverage to their citizens could unlock value equivalent to 3 to 13 percent of GDP by 2030.
iProov secures the onboarding and authentication of digital identities through science-based face biometrics – so people have easier online access to online services, while organizations can pursue digital transformation strategies as securely as possible.
That doesn’t sound a technology intended for restricted, non-governmental purposes.
Not an endorsement
As I wrote about previously, when it comes to demographics and bias in AI facial comparison, the accuracy of the algorithms per se is not the entire story– especially if that accuracy is used to promote the efficacy of the algorithms in non-ideal conditions– aka, perhaps the face isn’t straight-on, the lighting isn’t good, the subjects are moving slightly (such as babies and/or parents trying to wrangle babies), or the quality of the camera isn’t great.
The term for photos not taken under these ideal conditions is “in the wild,” but those subjects typically don’t even know they’re being photographed. So “selfies” and “video selfies” taken by migrants using their phone cameras exist in a kind of weird limbo between “ideal” and “in the wild,” or “passport photo” vs. “caught on surveillance video while shoplifting.”
In 2019, NIST performed testing as part of its Face Recognition Vendor Test (FRVT) program looking specifically for “demographic effects” on facial recognition algorithms, in which they noted “demographic effects even in high-quality images, notably elevated false positives. Additionally, we quantify false negatives on a border crossing dataset which is collected at a different point in the trade space between quality and speed than are our other three mostly high-quality portrait datasets.”
In a 1-1 verification comparison, a false negative would be a failure to recognize that two photos show the same person, whereas a false positive would be a failure to recognize that they don’t show the same person.
The report found that “false positive differentials are much larger than those related to false negatives and exist broadly, across many, but not all, algorithms tested. Across demographics, false positives rates often vary by factors of 10 to beyond 100 times. False negatives tend to be more algorithm-specific, and vary often by factors below 3.”
The report only looked at false negatives for border crossing photos, but noted that in those “lower-quality border crossing images, false negatives are generally higher in people born in Africa and the Caribbean, the effect being stronger in older individuals.” Those images were considered “lower-quality,” attributable to being “collected under time constraints, in high volume immigration environments. The photos there present classic pose and illumination challenges to algorithms.”
Be that as it may, NIST also described them as “collected with cameras mounted at fixed height and are steered by the immigration officer toward the face.” In other words– this was a scenario in which CBP officers took photos using their own cameras and compared those images to passports etc. of documented pedestrian travelers crossing the border. Even then, the report says “We don’t formally measure contrast or brightness in order to determine why this occurs, but inspection of the border quality images shows underexposure of dark skinned individuals often due to bright background lighting in the border crossing environment.”
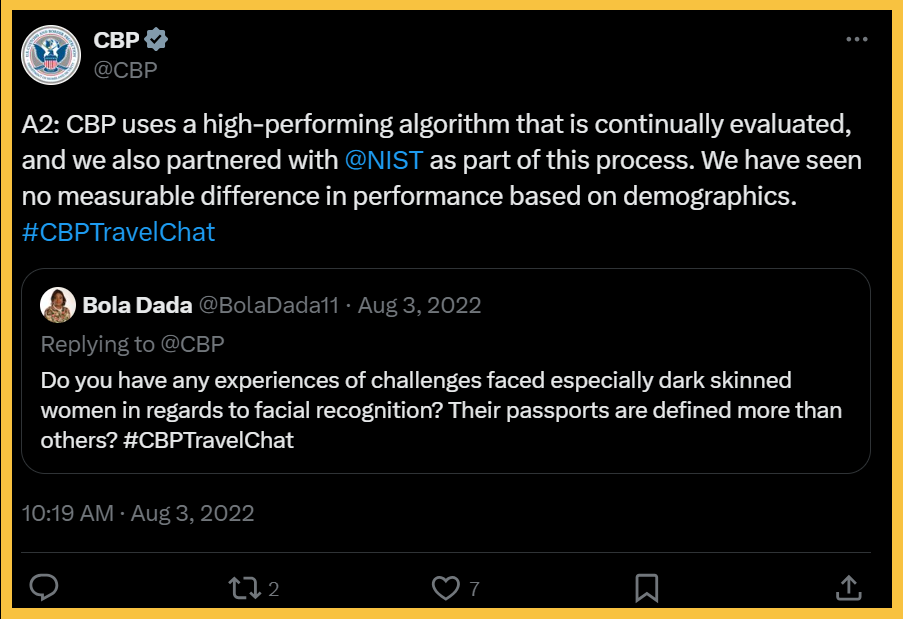
And yet when asked about demographic effects on facial recognition, CBP’s typical response is to cite the 2019 NIST study showing that the NEC-3 algorithm (CBP switched to this algorithm in 2020) was 97% accurate, an assessment based on number of false positives in a 1-to-many identification comparison based on photos taken from air travelers or pedestrian travelers as compared to a flight manifest built from “exit” photos of the same people pictured in the “entry” photos.
The question of whether there’s meaningful bias in an algorithm really comes down to the context in which it will be used, and– critically– the context of this research differs, in nearly every way, from the experience of migrants trying to use the CBP One app.
Is the NEC-3 algorithm good at recognizing when there is one (and only one) photo of you in a collection of images taken of you when you leave the country by airplane that matches the photo of you when entered it? Answer: Yes, really good– under ideal conditions.
‘CBP believes that the December 2019 NIST report supports what we have seen in our biometric matching operations—that when a high-quality facial comparison algorithm is used with a high-performing camera, proper lighting, and image quality controls, face matching technology can be highly accurate,’ the spokesperson said.
CBP Is Upgrading to a New Facial Recognition Algorithm in March
To be fair, that spokesperson was not talking about CBP One. Development of CBP One hadn’t even been announced in February, and wouldn’t be announced until August. For that matter, nearly all discussion by CBP of their use of AI facial recognition isn’t about CBP One– and that’s the problem.
How about recognizing whether you’re the same person in a photo captured now as you were in a previous photo, and a least one of those photos was taken by yourself using an app on your phone and therefore very likely to be of “low quality,” and neither of them are taken from travel documents because you have no travel documents, and you’re (let’s say) a dark-skinned elderly woman?
You can’t meaningfully vouch for the accuracy and the lack of “demographic effects” in an algorithm that is used in a way explicitly differing from every scenario in which you’ve previously tested that technology. That is, by far, the most frustrating thing about trying to investigate complaints about CBP One’s performance when it comes to facial comparison and liveness detection.
The same spokesperson continued:
CBP’s operational data demonstrates that there is virtually no measurable differential performance in matching based on demographic factors. In instances when an individual cannot be matched by the facial comparison service, the individual simply presents their travel document for manual inspection by an airline representative or CBP officer, just as they would have done before.
In the context of CBP One, that’s like saying “The door isn’t broken. But if it is, you can come in through the window. Except the window has razor wire on it.”
Both false negatives and false positives could play into the causes of error rates in CBP One’s facial recognition. But false positives in a 1:n comparison are the errors more commonly discussed, which I suspect is because that’s the scenario in which you’re concerned about detecting imposters. You’re comparing an image of one person to a gallery of images, and designing an algorithm to avoid the error of incorrectly identifying this person as one of those people– because imposters are people who can pass as members of a group when they’re not. False negatives, on the other hand, occur in 1:1 matching when photo quality is low, and the camera can’t recognize certain skin colors or faces under strange lighting. I.e., the kinds of problems afflicting users of CBP One.
Charles Romine, former director of NIST’s Information Technology Laboratory, noted in 2020 that “False positives might pose a security concern to the system owner, as they may allow access to imposters.” In this case the system is the United States, and the imposters are migrants pretending to people allowed to enter the country legally.
Alive and recognized
“Liveness detection” is another, newer, way to detect imposters.
It isn’t about telling whether you’re alive or dead, as it might sound, but more like a selfie-as-CAPTCHA. It’s trying to distinguish between an image taken of you right now and, say, a previously-taken picture of you that has been uploaded. It’s also called “Presentation Attack Detection,” in case its application to detecting imposters isn’t clear enough. As iProov’s website puts it,
iProov’s patented Flashmark technology is the only solution in the world to defend against replay attacks as well as digital and physical forgeries, and has been adopted by many Governments [sic] and financial institutions.
iProov’s World-Leading Dynamic Liveness Deemed State of the Art by NPL
So you could say that Facial Comparison is “I see your face, and it’s definitely your face,” or “I see your face, and have matched it to someone in a gallery of other faces,” while PAD is “I see your face, and not just someone holding up a picture of your face.”
As said in the previous post, I suspect that at least some of the accusations of bias are actually in response to liveness detection, not facial recognition. Liveness detection is susceptible to the same biases as FRT which, according to the biometric company IDR&Ds website, means that accuracy is “essential where facial recognition is used for unsupervised security processes.” The company has worked to counter demographic bias, both directly and by correcting for subfactors that cause such effects.
One example, the site points out, “is persons wearing hijabs, which is highly correlated with gender and ethnicity and also directly affects the performance of the facial liveness system.”
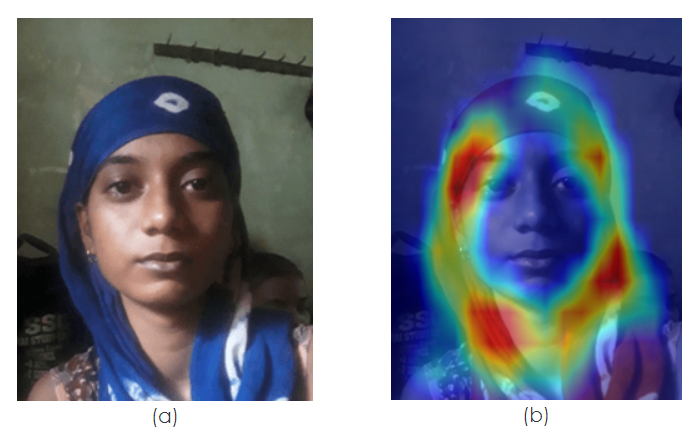
“For categories where algorithms underperformed, teams analyzed images in the dataset to determine the areas of the image deemed ‘important’ when making its prediction. This method helps determine discriminating areas in the image.”
The caption on this image (left) reads “Heat maps of neural network demonstrate the area of interest of the facial anti-spoofing algorithm: a) original image of a woman in the national headdress, b) heat map of neural network has higher attention on the medium and bottom parts of the headdress“
Photo cedit: ID R&D Biometric Authentication
The algorithm is looking everywhere but the face, in this example. The focus is on the hijab, which is something of an irony when compared to cultural attitudes– even a machine can’t help but focus on the head covering, to the exclusion of the person wearing it.
Untrained eyes
A GAO report published in September 2023 noted the stunning lack of training for DHS officers on facial recognition across DHS agencies, including CBP. CBP had been using facial recognition since 2018, about the same duration as the FBI, but (like the FBI) had never implemented a training requirement. Beyond that, GAO found that CBP didn’t even track the number of facial recognition searches that staff conducted.
Six agencies with available data reported conducting approximately 63,000 searches using facial recognition services from October 2019 through March 2022 in aggregate—an average of 69 searches per day. We refer to the number of searches as approximately 63,000 because the aggregate number of searches that the six agencies reported is an undercount. . .CBP) did not have available data on the number of searches it performed using either of two services staff used.
FACIAL RECOGNITION SERVICES: Federal Law Enforcement Agencies Should Take Actions to Implement Training, and Policies for Civil Liberties,
A footnote read: “CBP officials were unable to provide information on the number of facial recognition searches staff conducted during this time because neither the agency nor the services tracked this information.”
It’s clear from those numbers that we’re not talking about searches for migrants, or at least not searches conducted for migrants in the same way that TVS consults databases. CBP officers are not performing an average of 11.5 searches a day for the same information that the CBP One app checks for thousands of migrants.
Even though CBP didn’t give its numbers, and even though they didn’t track the number of searches (which isn’t good), it would make zero sense for those all to be covert searches conducted manually. For one thing, the CBP One app launched in 2020 and was immediately put to work doing that specific task. For another, the report describes these agencies as searching using specific facial recognition services, and for CBP those were IntelCenter (“to search photos against a gallery of over 2.4 million faces extracted from open-source terrorist data”2) and Marinus Analytics (“to identify victims of human trafficking”).
(I was almost disappointed to find that CBP was not listed as consulting Clearview.AI,3 the facial recognition company that was caught scraping user images and information from Facebook, and has been sued by multiple countries for violating the privacy expectations of their citizens (the U.S. isn’t so protective of such things). However, all of the other five agencies did– the FBI, the Bureau of Alcohol, Tobacco, Firearms, and Explosives, the DEA, the U.S. Marshals Service, Homeland Security Investigations (ICE4), and the U.S. Secret Service.)
CBP maintains that cracking down on trafficking (especially when it means rescuing kids caught up in it) plays a major role in its use of FRT, and countering terrorism is IMO the best argument for having a Department of Homeland Security in the first place. But whether those are legitimate uses for FRT or not, these are cases of CBP officers performing the searches. Untrained officers apparently, but still– human beings making decisions and evaluations, which is the reason they need training.
Biased toward automation
Algorithms are trained, too, but we don’t get to see it. The possibility that an algorithm has been trained exclusively or primarily on images of white men is one explanation for demographic bias displayed by that algorithm– NIST proposed it as a reason for false positives, as mentioned above. But false negative errors are usually a result of poor photo quality/lighting, and those a problems even when there is a CBP officer taking the photo. When there’s no CBP officer taking the photo, no CBP officer looking at the photo, and no CBP officer making the decision to reject the photo– and by extension, the migrant in the photo– there’s no place where a human steps in, in that scenario. Not even to manually verify an individual’s identity after an algorithm fails to do so.
The “black box” nature of artificial intelligence means we don’t get direct access to the decision-making process– because there is no decision-making process, per se (of course I say this now, but probably next week there will be). But we can look at the decisions made by the algorithm and notice whether they’re correct or incorrect, which is the benefit of NIST’s ongoing facial analysis testing.
CBP is also a black box, in that (for the most part) it doesn’t inform the public when it makes changes to the app. It doesn’t provide release notes. It doesn’t say what to do if the app isn’t working, except to email CBPOne@cbp.dhs.gov (I tried that but received no reply, so I joked in the last post about needing to submit a FOIA request for tech support).
This is why I wonder who notices when TVS makes an error– of any kind– and what they do about it. In person, an officer can step in and tell the computer that you are, in fact, yourself. Who contradicts the computer in the app? What record is there when an error occurs, and who sees it, and what do they do about it? None of us are exactly fond of getting to talk to a “robot” when we call a business, but some people are dead set on getting “a real person”– and they’re only trying to contest their water bill. But if talking to the robot is the only way to do that, then the choices are: a) talk to the robot, or b) don’t, and nothing changes.
CAPTCHAs always ask you to prove you’re not a robot, but CBP One is a robot asking you to prove much more than that. In addition to your personal details, your face is the only evidence you can provide. And if CBP One doesn’t accept that, well, nothing changes.
- They also appear to be unaware that police in the United States have used Clearview FRT on American citizens for nearly a million searches, according to Clearview, to compare their faces to Clearview’s database containing 30 billion images (again, according to Clearview founder and CEO Hoan-Ton-That). ↩︎
- The nature of “open-source terrorist data” is information “only available to government users in intelligence, military and federal law enforcement.” ↩︎
- Reading recommendation: Your Face Belongs to Us: A Secretive Startup’s Quest to End Privacy as We Know it, by Kashmir Hill. ↩︎
- See American Dragnet: Data-Driven Deportation in the 21st Century, a massive report from a two-year investigation into ICE’s surveillance activities and their impacts on both immigrants and civilians. ↩︎